When Man Group chief executive Luke Ellis discusses his investment company’s results with analysts he chooses his words carefully. He knows better than most that the machines are listening.
The crown jewel of Man is its $39bn hedge fund group AHL, whose algorithms scour huge data sets for profitable signals that feed into investment decisions.
One of the hottest areas in this field is “natural language processing”, a form of artificial intelligence where machines learn the intricacies of human speech. With NLP, quant hedge funds can systematically and instantaneously scrape central bank speeches, social media chatter and thousands of corporate earnings calls each quarter for clues.
As a result, Mr Ellis’s quant colleagues have coached him to avoid certain words and phrases that algorithms can be particularly sensitive to, and might trigger a quiver in Man’s stock price. He is much more careful about using the word “but”, for example.
“There’s always been a game of cat and mouse, in CEOs trying to be clever in their choice of words,” Mr Ellis says. “But the machines can pick up a verbal tick that a human might not even realise is a thing.”
This is a growing phenomenon. Machine downloads of quarterly and annual reports in the US — scraped by an algorithm rather than read by a human — has rocketed from about 360,000 in 2003 to 165m in 2016, according to a recent paper by the US’s National Bureau for Economic Research. That was equal to 78 per cent of all such downloads that year, up from 39 per cent in 2003.
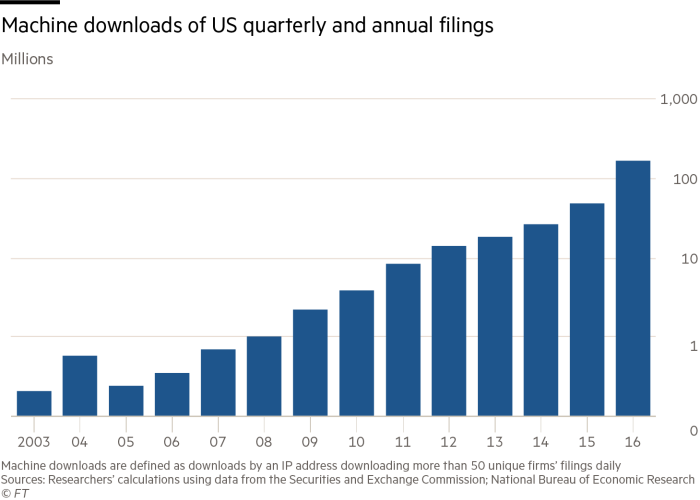
The paper — How to Talk When a Machine Is Listening: Corporate Disclosure in the Age of AI — points out that companies are keen to show off their business in the best possible light. They have steadily made reports more machine-readable, for example by tweaking the formatting of tables, as a result of this evolving analysis.
“More and more companies realise that the target audience of their mandatory and voluntary disclosures no longer consists of just human analysts and investors,” authors Sean Cao, Wei Jiang, Baozhong Yang and Alan Zhang note. “A substantial amount of buying and selling of shares are triggered by recommendations made by robots and algorithms which process information with machine learning tools and natural language processing kits.”
However, in recent years the corporate adjustment to the reality of algorithmic traders has taken a big step further. The paper found that companies have since 2011 subtly tweaked the language of reports and how executives speak on conference calls, to avoid words that might trigger red flags for machine listening in.
Not coincidentally, 2011 was when Tim Loughran and Bill McDonald, two finance professors at the University of Notre Dame, first published a more detailed, finance-specific dictionary that has become popular as a training tool for NLP algorithms.
Since 2011, words deemed negative in the Loughran-McDonald dictionary have fallen markedly in usage in corporate reports, while words considered negative in the Harvard Psychosociological Dictionary — which remains popular among human readers — show no such trend.
Moreover, using vocal analysis software, the authors of the National Bureau for Economic Research paper found that some executives are even changing their tone of voice on conference calls, in addition to the words they use.
“Managers of firms with higher expected machine readership exhibit more positivity and excitement in their vocal tones, justifying the anecdotal evidence that managers increasingly seek professional coaching to improve their vocal performances along the quantifiable metrics,” the paper said.
Some NLP experts say some companies’ investor relations departments are even running multiple draft versions of releases through such algorithmic systems to see which scores the best.
One word can say a lot . . .
Positive:
Proactively
Satisfying
Revolutionise
Negative:
Aggravate
Restated
Bottleneck
Uncertainty:
Anomaly
Appears
Clarification
Litigation:
Affidavit
Felony
Litigation
Source: Loughran-McDonald dictionary
“Access to NLP tools has become an arms race between investors and management teams. We see corporates increasingly wanting to have access to the same firepower that hedge funds have,” says Nick Mazing, director of research at Sentieo, a research platform. “We are not far from someone on a call reading ‘we said au revoir to our profitability’ versus ‘we recorded a loss’ because it reads better in some NLP model.”
However, Mr Mazing said that NLP-powered algorithms are also continuously adjusted to reflect the increasing obfuscation of corporate executives, so it ends up being a never-ending game of fruitless linguistic acrobatics.
“Trying to ‘outsmart the algos’ is ultimately futile: buyside users can immediately report sentence misclassifications back to the model so any specific effort to sound positive on negative news will not work for long,” Mr Mazing says.
Indeed, most sophisticated NLP systems do not rely on a static list of sensitive words and are designed to both identify problematic or promising combinations of words and teach themselves a chief executive’s idiosyncratic styles, Mr Ellis notes. For example, one CEO might routinely use the word “challenging” and its absence would be more telling, while one that never uses the word would be sending as powerful a signal by doing so.
Machines are still unable to pick up non-verbal cues, such as a physical twitch ahead of an answer, “but it’s only a matter of time” before they can do this as well, Mr Ellis says.
Twitter: @robinwigg